The technology enables users to convert several 2D images into magnificent realistic 3D environments. Neural radiance fields (NeRF) represent the newest groundbreaking technology that transforms the way we handle computer vision and graphics. Through this article, you will understand how neural networks function in NeRF to produce accurate novel views of complex scenes.
NeRF Meaning 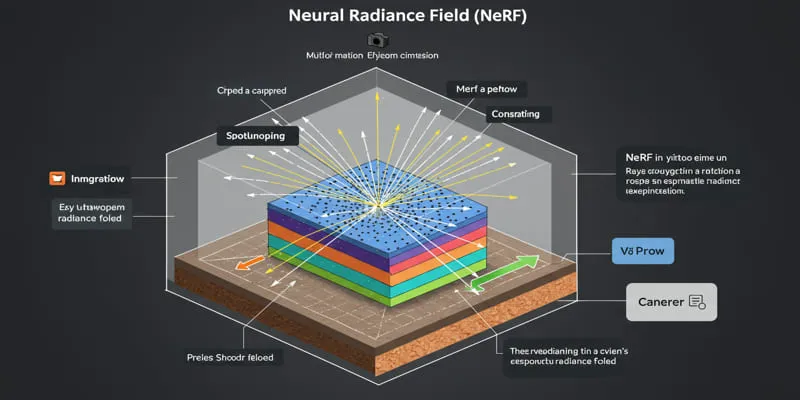
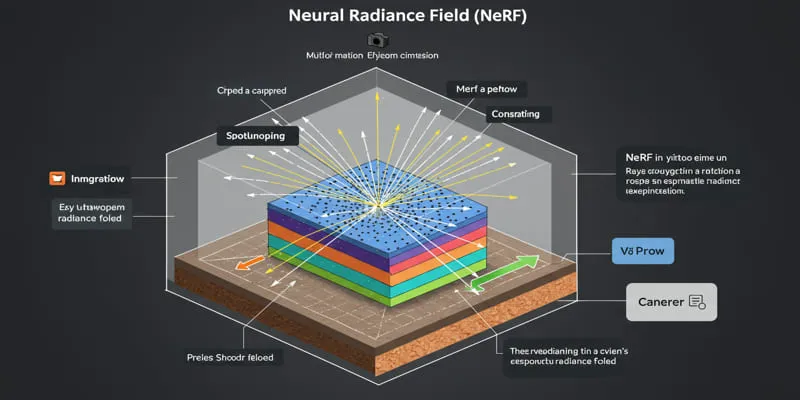
Neural Radiance Field, also known as NeRF, marks a transformational
development within computer vision and graphics. The innovative method
transforms sets of 2D images into photorealistic 3D scenes, revolutionizing
the process of rendering complex environments. ## The Fundamentals of NeRF The
fundamental operation of NeRF relies on neural networks to both acquire and
present three-dimensional spaces. NeRF establishes 3D space reconstruction
along with geometry and appearance through training on varied scene images
taken from various points of view. NeRF surpasses standard 3D modeling with
its capability to produce highly detailed realistic visuals. ## How NeRF Works
The neural network of NeRF incorporates all elements of a 3D scene. A 3D
coordinate pair and a selected viewing direction produce predictions from the
network concerning color and density output. Each pixel request to the network
lets users produce new viewpoints in desired images. NeRF achieves its magic
through its capability to generate smooth transitions by using known views to
precisely fill in missing information. NeRF software generates precise 3D
models which practitioners can view regardless of the initial input imagery’s
available perspectives. ## The Science Behind NeRF: How It Works Neural
Radiance Fields (NeRF) offers a revolutionary method for creating 3D scene
reconstruction and generating novel views. The NeRF neural network functions
as a volumetric scene function model that acquires geometry and appearance
characteristics. ### Neural Network Architecture The NeRF model employs a
multi-layer perceptron (MLP) as its primary architecture. The network accepts
a 5D coordinate (x, y, z, θ, φ) indicating a 3D position along with viewing
direction for input data and generates the volume density results together
with view-dependent emitted radiance values at that point. ### Ray Casting and
Volume Rendering NeRF generates new view perspectives through its
implementation of ray casting procedures. A ray travels through the entire
scene at every pixel position that needs to be rendered. The network obtains
density and color information from the MLP through point queries that occur at
different locations along the specified ray. The volume rendering equations
combine these retrieved values to generate pixel final colors. ### Training
Process NeRF requires a set of images together with their camera position data
while it trains itself. A learning paradigm exists where the system works to
decrease its output regressions against actual image references. The training
process enables NeRF to learn the 3D scene structure and appearance
automatically with no need for direct 3D supervision. ### Positional Encoding
The positional encoding method represents a main breakthrough in NeRF
development. The combination of directional coordinates with sinusoidal
functions in higher dimensional space enables NeRF models to represent both
smooth and high-frequency elements in the scene while reconstructing it more
accurately. ## Practical Applications of NeRF Technology The fast
development of Neural Radiation Fields (NeRF) technology has moved from
theoretical origins to operational status, serving multiple practical uses.
Academic institutions and professional sectors utilize this technology because
it enables detailed 3D model creation from standard two-dimensional imaging
sources. ### Virtual Reality and Gaming NeRF represents an advancement that
enables the development of truly immersive virtual spaces. Game developers can
create realistic 3D environments by using few source pictures, cutting
expenses and shortening production cycles. This technology also makes
expansive photorealistic game worlds possible, allowing players to navigate
authentic worlds with exceptional detail. ### Architecture and Real Estate
NeRF provides modern approaches for constructing real estate visualizations
within architectural spaces. The combination of several images enables
realtors to develop virtual property tours that bring accurate remote
exploration possibilities to potential buyers. The use of NeRF technology
enables architects to develop realistic 3D renderings of their designs
starting from their conceptual sketches. ### Film and Visual Effects Because
NeRF provides exceptional capabilities in visual effect creation and virtual
set design, the film industry has widely adopted its applications. Film
productions can attain uninterrupted camera operations and unrealistic camera
angles through new viewing syntheses from original footage reduction and CGI
avoidance. The technology brings unmatched benefits to construction of
realistic digital actor duplicates as well as historic environment
restorations which appear authentic. ## Advantages and Limitations of NeRF ###
Key Advantages Neural Radiance Fields (NeRF) offer several compelling benefits
in the realm of 3D scene representation. Fundamentally, NeRF generates the
most detailed and realistic novel views available. NeRF learns a continuous
volumetric scene function that enables it to produce precise realistic
visualizations even when the viewing angle was absent in the training process.
Notable Limitations NeRF offers its users strong features but also certain
drawbacks. The high computational power needed for both training operations and final rendering operations represents a principal drawback in NeRF implementation. A NeRF model requires long periods of optimization, which extend into multiple hours or complete days based on the scene complexity. The performance of NeRF systems deteriorates when operating in situations containing changing lighting conditions together with dynamic moving objects. The model produces faulty results when it encounters scenes that remain unchanging and have illumination that stays identical because these assumptions get broken during operation. ## The Future of NeRF: Emerging Trends and Developments Neural Radiance Fields (NeRF) are experiencing significant advancements through various interesting developments in their evolution process. New developments will allow NeRF applications to grow in multiple industrial sectors. ### Real-time Rendering and Interactivity The advancement of NeRF technology focuses on achieving real-time rendering as one of its most exciting breakthroughs. The scientific community focuses on enhancing NeRF algorithms while utilizing high-performance GPUs to produce faster renderings. Interactive NeRF experiences will become possible through this development because users will be able to move freely within generated 3D settings in real time. ### Integration with AR and VR Technologies The combination between NeRF technology and Augmented Reality (AR) and Virtual Reality (VR) systems shows great potential in the field. The combination of these technologies would bring disruptive changes to immersive experiences by producing more authentic and responsive virtual environments. You can experience historic sites or future architectural structures at an unparalleled level of detail while using their interactive features. ## Conclusion Neural radiance fields work as an innovative solution to develop 3D scene reconstruction with dynamic view synthesis capabilities. The deep learning technique utilizes NeRF to reconstruct photorealistic 3D images by processing limited 2D image datasets.