Generative AI has significantly advanced beyond basic text creation. By 2025, generative artificial intelligence for data scientists will unlock new opportunities, facilitating deep insight discovery, model building, and automated data analysis. As companies accumulate vast amounts of data, smarter tools become essential for data scientists. Generative AI develops intricate machine-learning models, reducing the need for extensive human intervention. It generates synthetic data for enhanced training and speeds up data wrangling.
Combining data science with creativity fosters innovation in both industry and research. Advanced AI models enable data scientists to maximize every phase of prediction and analysis. Generative AI serves as a key ally in data science processes. This article explores the practical applications, benefits, challenges, and future prospects of advanced generative AI solutions.
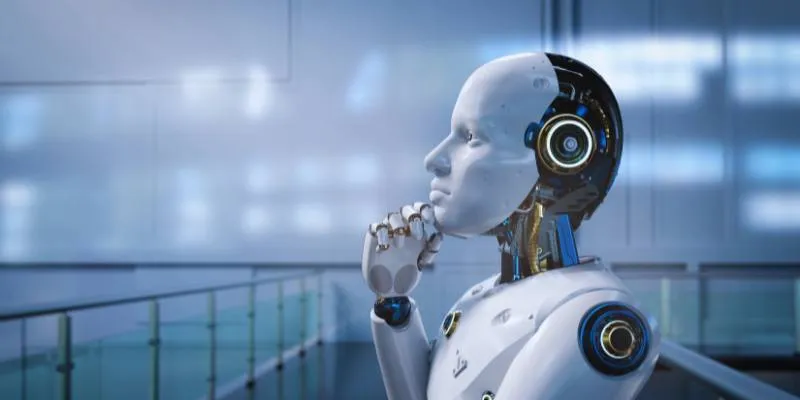****
Unlocking the Potential of Generative AI for Data Scientists in 2025
The role of data scientists is being transformed by generative AI. Today, it is not just a tool for creativity; it automates processes and helps solve complex data challenges in 2025. Generative AI can enhance dataset quality, fill in missing values, and clean cluttered data. Often, data scientists spend long hours preparing data for analysis. With intelligent preprocessing, generative AI accelerates this stage by automatically applying transformations and learning from trends, allowing valuable insights to emerge from raw data more quickly.
Generative AI also aids in feature engineering, identifying valuable data attributes without human error through automation. This leads to stronger models with less bias and improved generalization. Additionally, generative AI produces synthetic data, which is particularly useful when data is limited, sensitive, or biased. It generates diverse and balanced datasets, thereby strengthening integrity and resilience. Data scientists discover powerful, innovative approaches to validate models and test ideas.
Key Applications of Generative AI in Data Science Workflows
By 2025, data scientists will integrate generative AI into several stages of their workflows. Data collection and preparation are foundational. Generative AI autonomously searches through unstructured data sources, structures useful information, and organizes text responses into structured data categories, especially when dealing with survey answers. It also identifies sentiment, keywords, and trends, preparing data for further analysis without human labeling.
In the modeling phase, generative AI acts like an assistant by suggesting algorithms based on prediction goals and data characteristics. It provides automatically generated baseline models to data scientists, saving initial setup time. Furthermore, generative AI accelerates hyperparameter tuning by predicting optimal combinations based on past research, resulting in well- performing models faster than traditional trial and error.
Benefits of Generative AI for Data Scientists in 2025
Generative AI offers numerous advantages for data scientists, enhancing the speed and impact of their work. Automation is a clear benefit, as repetitive tasks such as data cleansing, gap filling, and report generation become routine, freeing up time for deeper investigation. Additionally, generative AI enhances creativity by suggesting new ideas, designs, and tools, inspiring data scientists and fostering innovation.
Another significant advantage is speed. Projects move from concept to delivery more rapidly as generative AI generates code snippets, baseline models, and prepares reports. This enables companies to adapt to changing needs more effectively. Moreover, error reduction is crucial. Human errors are common in manual data preparation, but standardized rules applied by generative AI improve data quality and model reliability.
Challenges and Ethical Concerns in Generative AI Adoption
Despite its benefits, generative AI presents challenges and risks. Data bias is a primary concern, as generative AI can replicate and amplify biases present in training data, leading to unfair or inaccurate models. Transparency is another complex issue, as many generative AI models operate as black boxes, making it difficult for data scientists to justify their conclusions, complicating regulatory compliance, especially in finance and healthcare.
Data privacy is also a concern, as generative AI often relies on access to sensitive data for training. Strict safeguards are essential to mitigate privacy risks. Although synthetic data needs thorough validation, it can be beneficial. Job displacement is another worry for some experts; as automation increases, routine data tasks disappear. Data scientists must adapt by focusing on strategy, interpretation, and communication rather than manual labor.
Future Trends: Generative AI’s Evolving Role in Data Science
Generative AI continues to evolve in data science. Personalized AI assistants are a notable development, learning from the preferences, methods, and projects of individual data scientists, thereby accelerating work further. Multimodal AI is an exciting trend, as future generative AI integrates text, images, videos, and sensor data, opening new possibilities in industries such as manufacturing, retail, and healthcare, while also enhancing explainability.
Future generative models will offer transparent reasoning, building trust among authorities and stakeholders. Collaborative AI is becoming common, allowing teams to work together using generative models in shared environments where everyone can view produced code, comments, and ideas, enhancing knowledge-sharing and collaboration. Lastly, self-healing AI pipelines are emerging. Generative AI monitors data flows, identifies issues, and automatically suggests corrections, maintaining analytics pipelines and reducing downtime. With these advancements, generative AI for data scientists evolves from a useful tool to an indispensable ally.
Conclusion:
Generative AI has advanced beyond text generation. By 2025, it will transform data science processes entirely, improving accuracy, speed, and creativity from data preparation to model creation and reporting. However, challenges related to ethics, transparency, and bias remain. Successful adoption requires human oversight and balanced governance. Even smarter, more transparent, and collaborative tools are on the horizon. For data scientists ready to embrace these advances, innovative AI tools offer new opportunities to create value across sectors.